Wegene Negese, an MSc student at Arba Minch University (Climate) and employee of the Ethiopian Meteorology Institute (EMI), has conducted validation measurements of the low-cost sensor system with SPS30 Sensirion at the EMI meteorological stations of Addis Ababa and Adama. For a period of four months, he collocated the sensor system with itself, and conducted gravimetry measurements. Gravimetry is the reference method for calibrating PM2.5 measurement instruments.
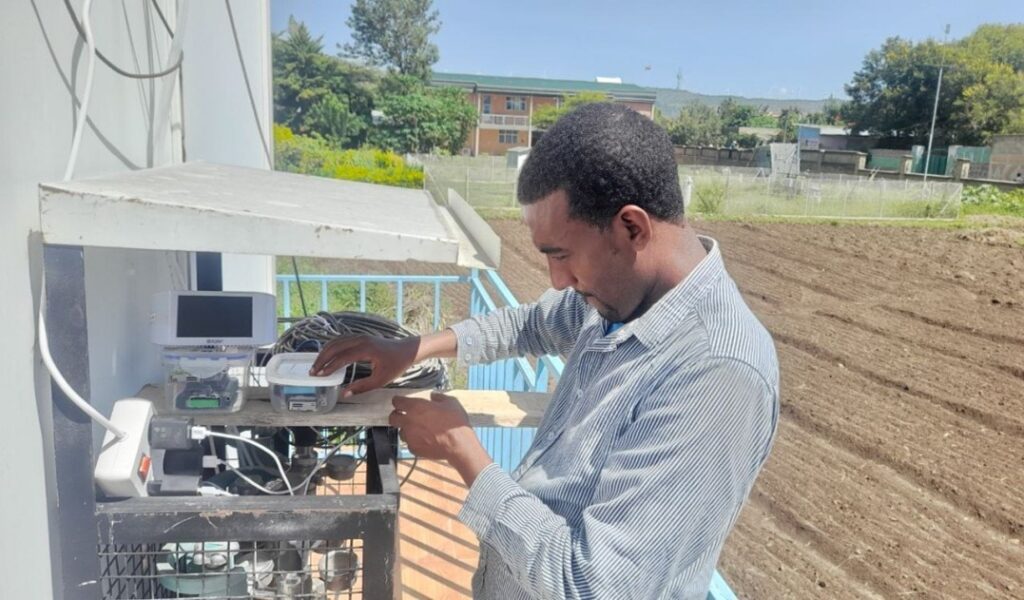
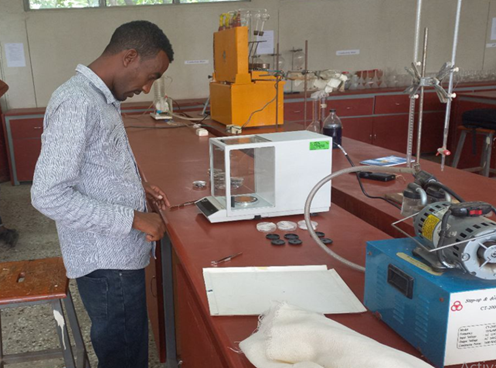
He is currently working on his MSc thesis, but I can already present some preliminary results:
- The coefficient of variation (CV; a measure of variation between two identical instruments) was 9.5% for two sensor systems in Addis Ababa (based on 12,677 10-minute averages), and 4.4% for two sensor systems in Adama (based on 4,135 10-minute averages). This indicates that the variation between two sensor systems is lower than 10%. 10% is set as a maximum allowed CV for measurement instruments by the NIOSH and the US EPA.
- The sensor system systematically measures lower than gravimetry, but the correlation is strong. Linear regression of all data points of Addis Ababa and Adama combined (n=16) leads to a slope of 1.62 with an R2 of 0.99. The Pearson correlation is 0.97.
The data of Wegene confirms wat I found in earlier data with measurements in Arba Minch: the SPS30 Sensirion has low within-variation, and shows a stable bias both under ambient and indoor (high) concentration settings versus gravimetry measurements (see this publication). In other words: the SPS30 Sensirion appears to be a very good sensor under Ethiopian circumstances.